This feature first appeared in the Spring 2015�issue of Certification Magazine. Click here to get your own print or digital copy.
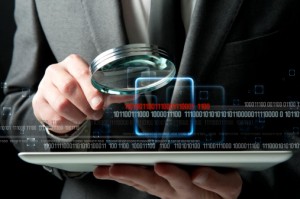
Big Data is one of the "biggest" buzzwords to hit both businesses and IT shops over the past decade. Analysts and researchers predict that Big Data analytics will contribute toward significant challenges, ranging from curing disease to assessing market trends. At the same time, Big Data will pose challenges and opportunities for information security professionals. Security personnel who embrace this trend early will find themselves well positioned to manage Big Data as a strategic asset for both the business and its IT personnel.
Information security teams should plan to address two significant questions related to Big Data operations. First, what security implications does the use of Big Data by the business raise? In many cases, IT security professionals will bear primary responsibility for securing the data sources and analysis tools used by Big Data operations.
Second, how can those same professionals leverage Big Data to improve the analytics supporting information security operations? Security professionals have long drowned in the multitude of data available to them and Big Data tools provide hope for new ways to pore through that data and find the needles in the haystack with significant security implications.
What is Big Data?
One of the first questions facing security professionals is fundamental to the conversation: What is Big Data anyway? The term means many different things to different audiences. Some use it to refer to the very large datasets generated by modern information systems and sensors. Others use it to describe the new analytic tools used to process that raw information into actionable intelligence. Yet another group applies the term Big Data to the output of those analytic processes. The reality is that all of these items are components of Big Data operations.
What makes a dataset Big Data? Is it gigabytes, terabytes or petabytes? There is no common consensus on a single threshold that qualifies a data source as "big," but most experts agree that Big Data embodies the "Three Vs":
Volume - While there is no numeric threshold for the size of the dataset, most analysts only call a dataset big if it is large enough that traditional analysis techniques won't yield useful results. If it fits in an Excel spreadsheet, it's not Big Data!
Variety - Big Data analytics leverage information from a wide variety of data sources. The true power of Big Data lies in correlation and that requires information from databases, sensors, physical processes and other diverse sources.
Velocity - Big Data moves quickly. It may arrive in torrential storms and/or change at a rapid rate. Analysis tools must be able to cope with this unprecedented velocity.
Using Big Data requires access to new tools designed to handle the mix of data sources that analysts encounter. Many types of Big Data come in very unstructured formats, such as social media postings, books, email messages and web pages. All of these sources contain a great wealth of information but it does not come in a predefined format that facilitates easy analysis. Other data sources may be semi-structured, using a flexible datalanguage such as XML or JSON to label attributes and values but not require any particular fields.
The tools and techniques used in Big Data analysis accept large quantities of unstructured and semi-structured data that arrive with velocity, variety and volume and process them into useful information. This analysis is difficult, but it may reveal new insights that went undiscovered using traditional analysis techniques on highly structured data.
Securing Big Data
From a security perspective, Big Data poses new challenges. Organizations performing Big Data analysis may have access to large quantities of extremely sensitive information. Think about some of the areas that are promising sources for Big Data analysis: individual health records, financial information, and location information. Companies collecting this data must protect it carefully to guard against the reputational, financial and ethical implications of a security breach.
One of the biggest conflicts that occurs during many Big Data implementations is striking the appropriate balance between security and access. Big Data efforts are most successful when many people throughout the organization have access to analysis tools and their output. Broad access to information facilitates the data-informed decision-making processes that deliver value from Big Data. At the same time, organizations may be justifiably hesitant to put sensitive information in the hands of large numbers of staff. As organizations navigate the waters of Big Data security, they must strike a balance between these competing goals.
Many organizations attempt to solve this issue by using role-based security. They first define broad roles that grant widespread access to large quantities of less sensitive information. These solve the data needs of the vast majority of users. They then create specialized roles for more sensitive data elements and grant access to those roles in a much more restrictive manner. This allows broad access to most information but retains tight control over the most sensitive data elements.
The second piece of the Big Data puzzle is implementing strong auditing practices. Businesses should log the use of data access privileges in a centralized log repository and then audit those logs periodically for inappropriate use. Security teams may use automated tools to assist with this, watching for unusual access patterns or access to extremely sensitive information. Audit logs also provide forensic capabilities, allowing investigators to determine who accessed sensitive information in the wake of a security breach.
Finally, organizations should perform routine permission audits that review the access rights granted to individuals. These audits should confirm that any resources accessed are deployed in their role. If the review detects any discrepancies, the reviewer should reconcile them with the individual's supervisor to determine whether continued access is appropriate.
Leveraging Big Data for Security
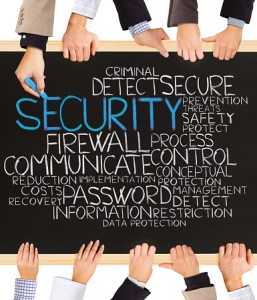
While security professionals should certainly focus on securing access to Big Data, they also may benefit from applying Big Data techniques themselves. After all, many security data sources create their own Big Data. Consider the vast quantities of logging information generated by firewalls, intrusion detection systems, access control systems, physical security systems and other pieces of security infrastructure. Security professionals know that important information likely resides within those logs, but lack the tools to unlock it and discover the few records with serious security ramifications.
For many years, intrusion detection systems (IDS) followed the straight-and-narrow path of signature detection. This technology worked quite simply � IDS vendors analyzed new threats and developed models of malicious activity known as signatures. Companies installed IDS on their networks and then regularly applied signature updates from vendors. The IDS sat on the network monitoring traffic and watching for activity that matched the patterns found in any of the signatures. When they detected a match, they flagged the records to administrators for further investigation.
Signature-based intrusion detection works well but has one fatal flaw: It is unable to detect novel attacks - intrusion by previously unknown methods - called "zero-day" events in security circles. If an intruder invents or acquires a previously unknown attack technique, he or she may use it with impunity until the IDS vendor discovers it, writes a new signature and applies the signature to IDS systems deployed around the world. This may result in days or weeks of lag time when intrusion detection systems remain blind to the attack, leaving networks vulnerable.
Big Data analysis techniques address this situation by supplementing tried-and-true signature detection techniques with more flexible anomaly detection techniques. In this approach, the IDS monitors traffic on the network and develops models of normal network activity. It may then watch future traffic and compare it to that model, looking for deviations from the norm.
For example, the IDS might trigger an alert when a custodial employee connects to the network from a foreign country because that doesn't match any prior patterns of activity. The same connection from the account of a salesperson who recently booked a flight to China may not arouse suspicion. This type of analysis requires the correlation of many unstructured data sources, such as personnel records, network logs and travel itineraries.
The flexibility of anomaly detection systems gives security organizations a fighting chance in the war against zero-day attacks. If the signature detection system fails to detect an intrusion, the anomaly detection system may still notice that the activity looks different from past network traffic and flag it for review.
Big Data poses interesting challenges and opportunities for information security professionals. Security personnel around the world should develop plans for how they will both secure their organization's Big Data repositories and, at the same time, leverage Big Data analysis techniques to improve the quality of their information security programs.
Important Update: We have updated our Privacy Policy to comply with the California Consumer Privacy Act (CCPA)