This feature first appeared in the Summer 2020 issue of Certification Magazine. Click here to get your own print or digital copy.
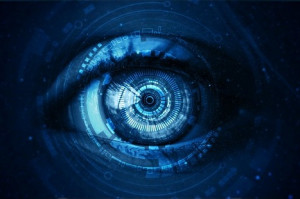
Artificial intelligence (AI) is an amazing realm and direction of research that promises to speed up the development of computer systems able to perform tasks that normally require human intelligence. In the bold future envisioned by AI, computers would have the capacity for visual perception, speech recognition, decision-making, translation between languages, and more.
Right now, the goal of keeping AI's impact on society beneficial motivates research in many areas, from economics and law to technical topics such as verification, validity, security, and control. This research is helping in so many ways, from an automation and processing standpoint to a security and Don't hack me or I think you are hacking me standpoint.
It may be little more than a minor nuisance if your laptop crashes or gets hacked. It becomes potentially a much bigger problem if your AI system glitches, or fall under remote control, and does something you would rather it not do: steers your car or airplane into harms way, turns off your pacemaker, freezes your automated trading system, shuts down your power grid.
Another short-term challenge is preventing a devastating arms race in lethal autonomous weapons. In the long term, an important question is what will happen if the quest for strong AI succeeds and an AI system becomes better than humans at all cognitive tasks.
As pointed out by I.J. Good in 1965, designing smarter AI systems is itself a cognitive task, done by humans. Such a system could potentially undergo recursive self-improvement, triggering an intelligence explosion leaving human intellect far behind. The actual self-aware stage — fearfully foretold in movies like The Terminator and The Matrix — could become a reality.
By inventing revolutionary new technologies, of course, such a superintelligence might help us eradicate war, disease, and poverty. Viewed in that light, the creation of strong AI might be the most consequential event in human history. Some experts have expressed concern, though, that it might also be the last — unless we learn to align the goals of the AI with ours before it becomes super intelligent.
The real, more troubling problem
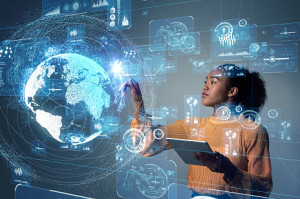
All of these hypothetical tangles pale in comparison to what may become the defining issue with AI: bias. The vast majority of AI research today is done by a mostly white male subset of the research population. A growing number of voices have begun to question what the effects might be of the core AI mindset being defined by a group with such a narrow worldview.
So how did we get here and where do we go from this point? At this stage, what kind of corrective actions could we take? Why is bias potentially harmful and what are the negative outcomes of bias as it relates to this situation? Is it acceptable for a strictly human-centered bias to guide the creation of AI, or should our goal be to remove bias altogether?
As I see it, gender and ethnic bias should not have a role in determining anything, except in studies through which you are working to eliminate it. I say this as a white male because I think that science make larger strides when pursued from a viewpoint where ethnicity and gender are eliminated completely.
We've already put AI in a variety of boxes, often via entertainment. In fiction, AI has had both male and female characteristics, has been both strongly sexualized and strictly asexual, has been viewed as both a self-actualized and controlling menace to human society and a parental caretaker overseeing periods of peace and prosperity.
In strictly fictional terms, I think the dominating AI that becomes self-aware and turns on its creators is generally a masculine concept, whether explicitly (think HAL 9000 from 2001: A Space Odyssey or Agent Smith from The Matrix) or implicitly (think Skynet from The Terminator).
AI generally becomes feminine when it is viewed as a servant (as seen via everything from The Jetsons mainstay Rosie the Robot to the ship's computer on Star Trek: The Next Generation) or sexualized in any way (as seen in films like Ex Machina or Her and on television via Westworld).
These examples are just entertainers sensationalizing AI for stories or the big screen. But when we look at real-world outcomes, at the research and actual creation of AI, the effect of such tropes is apparent. Apple's pioneering virtual assistant, Siri, has a pleasantly feminine voice. Though they aren't yet autonomous, so-called sex robots are already on the market. These are actual AI creations, not fantasy.
Biases skew outcomes
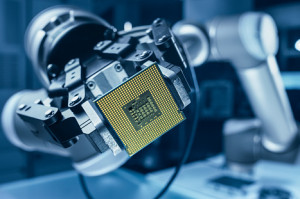
Bias, in all its forms, enters AI in much the same way as it does for all research, when a study group or control group is not selected from a wide enough variety of people, or across a group of people as widely dispersed, as is optimal. Also of concern is the the pool of respondents from whom representative views are drawn.
Properly formulating a pool of respondents in theory may seem like a no-brainer, but in practice it often leads to something called selection bias. When conducting a survey, for example, it's imperative to target a population that fits your survey goals. If you incorrectly exclude or include participants, then you may get skewed data results.
Usually this bias happens through lack of a clearly defined target population. Let's say a research team wants to limit its survey to people with low economic standing. This population could be defined in many ways: people who earn low salaries, people who lack disposable income, or people who have a low net worth after taking into account their property, income, and debt.
Each of these three descriptions could be successfully used to characterize the broad population you hope to reach. Each definition, however, is likely provide different results for your study. To avoid drawing information from the wrong people, the team has to be sure it creates a clear profile of the respondents needed to achieve research objectives before beginning the project.
Now imagine that the group making all of the decisions about our theoretical study is itself made up of similar individuals with identical (or nearly identical) perspectives. This is analogous to the current state of AI research. Even if best practices are being followed to formulate research activities and define the outcomes we're looking for, those formulas and definitions themselves are being given parameters by a highly select group.
Recent studies estimate that 80 percent of university professors studying AI are men, as are similar percentages of private sector researchers. Employees at leading tech companies are also largely white. A 2019 study, for example, found that just 2.5 percent of workers at Google are black, with Facebook and Microsoft faring only slightly better at 4 percent.
Necessary steps

At this stage of AI research and development, it will be hard but not impossible to balance existing bias. First, STEM and AI research teams need to actively recruit and hire outside of the norm the industry has established for itself. Companies and research organizations need to focus on recruiting and/or training and educating qualified women and non-whites to both participate in and lead research efforts.
To the extent that more equitable recruitment can be accomplished, it will winnow down two key forms of bias. First the authority bias, which favors opinions and ideas presented by authority figure within innovation teams.
Generally speaking, innovative ideas put forward by senior team members are preferred over all others, even if other inputs might be more creative and relevant to problem-solving.
With more diverse teams, there is less of a tendency to reflexively defer to the person in charge of the team. In mixed groups, people tend to be more willing to question authority and less likely to either formulate or defer to a group mindset.
Second is the loss-aversion bias. With this form of bias, once a decision has been made, people tend to defer to that decision rather than taking risks. This is driven both by fear of losing work done in preliminary and initial stages and by emotional investment in the original decision. Team members want to see things through.
In this situation, greater diversity makes it less likely that everyone will unite behind a single idea. Loss-aversion bias can also be remedied by what I like to think of as being the 11th commandment: Thou shalt not fall in love with thy solutions. I find professionally that it is possible to both love what my team comes up, and also embrace other compelling ideas from other teams.
Certain kind of biases are vital
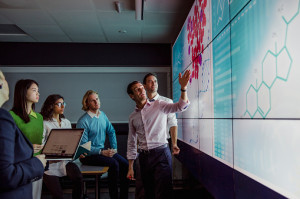
Is bias always bad? It depends on what you're asking about. Bias on the basis of gender or ethnicity is, of course, almost always harmful. In developing AI, the human predisposition to view certain situations as dangerous is a bias that is almost always helpful. And for certain kinds of questions, the only way to produce better answers is to be biased.
Many of the most challenging problems that humans solve are known as inductive problems — problems where the most correct or most beneficial answer cannot be definitively identified based on the available evidence. Finding objects in images and interpreting natural language are two classic examples.
An image is just a two-dimensional array of pixels — a set of numbers indicating whether locations are light or dark, green or blue. An object is a three-dimensional form, and many different combinations of three-dimensional forms can result in the same pattern of numbers in a set of pixels. Seeing a particular pattern of numbers doesn't tell us which of these possible three-dimensional forms are present: We have to weigh the available evidence and make a guess.
Likewise, extracting words from the raw sound pattern of human speech requires making an informed guess about the sentence a person might have uttered. The critical importance of this type of intelligence is the reason we need to incorporate certain patterns in AI but it has nothing to do with ethnicity or gender. The only way to solve inductive problems well is to be biased in favor of established systems and patterns.
Because the available evidence isn't enough to determine the right answer, you need to have predispositions that are independent of that evidence. And how well you solve the problem — how often your guesses are correct —depends on having biases that reflect how likely different answers are.
Humans are very good at solving inductive problems. Finding objects in images and interpreting natural language are two problems that people still solve better than computers. And the reason is that human minds have biases that are finely tuned for solving these problems.
Looking ahead
In some ways, bias is a larger problem than just what we find at the level of AI research and development. If we never attempt to eliminate ethnic and gender bias from all aspects of human society, then we aren't likely to ever be able to eliminate it from higher studies. There are numerous biases in existing educational systems, for example, that need to be flushed out before true equity can be established at higher levels.
In general, I don't think all forms of bias need to be, or ever can be, entirely eliminated. Since humans are as diverse as the AI we want to create, however, we need to deepen the diversity of our researchers and engineers. We need to seek to make all of humanity a representative set when we develop AI. This will only increase our chances of creating something that will benefit all of humankind.
Important Update: We have updated our Privacy Policy to comply with the California Consumer Privacy Act (CCPA)